The AWS Certified Machine Learning Specialty validates expertise in building, training, tuning, and deploying machine learning (ML) models on AWS.
Use this App to learn about Machine Learning on AWS and prepare for the AWS Machine Learning Specialty Certification MLS-C01.
Download AWS machine Learning Specialty Exam Prep App on iOs
Download AWS Machine Learning Specialty Exam Prep App on Android/Web/Amazon
[appbox appstore 1611045854-iphone screenshots]
[appbox microsoftstore 9n8rl80hvm4t-mobile screenshots]
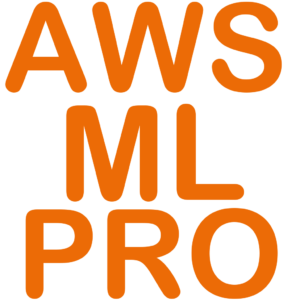
Download AWS machine Learning Specialty Exam Prep App on iOs
Download AWS Machine Learning Specialty Exam Prep App on Android/Web/Amazon
The App provides hundreds of quizzes and practice exam about:
– Machine Learning Operation on AWS
– Modelling
– Data Engineering
– Computer Vision,
– Exploratory Data Analysis,
– ML implementation & Operations
– Machine Learning Basics Questions and Answers
– Machine Learning Advanced Questions and Answers
– Scorecard
– Countdown timer
– Machine Learning Cheat Sheets
– Machine Learning Interview Questions and Answers
– Machine Learning Latest News
The App covers Machine Learning Basics and Advanced topics including: NLP, Computer Vision, Python, linear regression, logistic regression, Sampling, dataset, statistical interaction, selection bias, non-Gaussian distribution, bias-variance trade-off, Normal Distribution, correlation and covariance, Point Estimates and Confidence Interval, A/B Testing, p-value, statistical power of sensitivity, over-fitting and under-fitting, regularization, Law of Large Numbers, Confounding Variables, Survivorship Bias, univariate, bivariate and multivariate, Resampling, ROC curve, TF/IDF vectorization, Cluster Sampling, etc.
Domain 1: Data Engineering
Create data repositories for machine learning.
Identify data sources (e.g., content and location, primary sources such as user data)
Determine storage mediums (e.g., DB, Data Lake, S3, EFS, EBS)
Identify and implement a data ingestion solution.
Data job styles/types (batch load, streaming)
Data ingestion pipelines (Batch-based ML workloads and streaming-based ML workloads), etc.
Domain 2: Exploratory Data Analysis
Sanitize and prepare data for modeling.
Perform feature engineering.
Analyze and visualize data for machine learning.
Domain 3: Modeling
Frame business problems as machine learning problems.
Select the appropriate model(s) for a given machine learning problem.
Train machine learning models.
Perform hyperparameter optimization.
Evaluate machine learning models.
Domain 4: Machine Learning Implementation and Operations
Build machine learning solutions for performance, availability, scalability, resiliency, and fault
tolerance.
Recommend and implement the appropriate machine learning services and features for a given
problem.
Apply basic AWS security practices to machine learning solutions.
Deploy and operationalize machine learning solutions.
Machine Learning Services covered:
Amazon Comprehend
AWS Deep Learning AMIs (DLAMI)
AWS DeepLens
Amazon Forecast
Amazon Fraud Detector
Amazon Lex
Amazon Polly
Amazon Rekognition
Amazon SageMaker
Amazon Textract
Amazon Transcribe
Amazon Translate
Other Services and topics covered are:
Ingestion/Collection
Processing/ETL
Data analysis/visualization
Model training
Model deployment/inference
Operational
AWS ML application services
Language relevant to ML (for example, Python, Java, Scala, R, SQL)
Notebooks and integrated development environments (IDEs),
S3, SageMaker, Kinesis, Lake Formation, Athena, Kibana, Redshift, Textract, EMR, Glue, SageMaker, CSV, JSON, IMG, parquet or databases, Amazon Athena
Amazon EC2, Amazon Elastic Container Registry (Amazon ECR), Amazon Elastic Container Service, Amazon Elastic Kubernetes Service , Amazon Redshift
Important: To succeed with the real exam, do not memorize the answers in this app. It is very important that you understand why a question is right or wrong and the concepts behind it by carefully reading the reference documents in the answers.
Note and disclaimer: We are not affiliated with Microsoft or Azure or Google or Amazon. The questions are put together based on the certification study guide and materials available online. The questions in this app should help you pass the exam but it is not guaranteed. We are not responsible for any exam you did not pass.
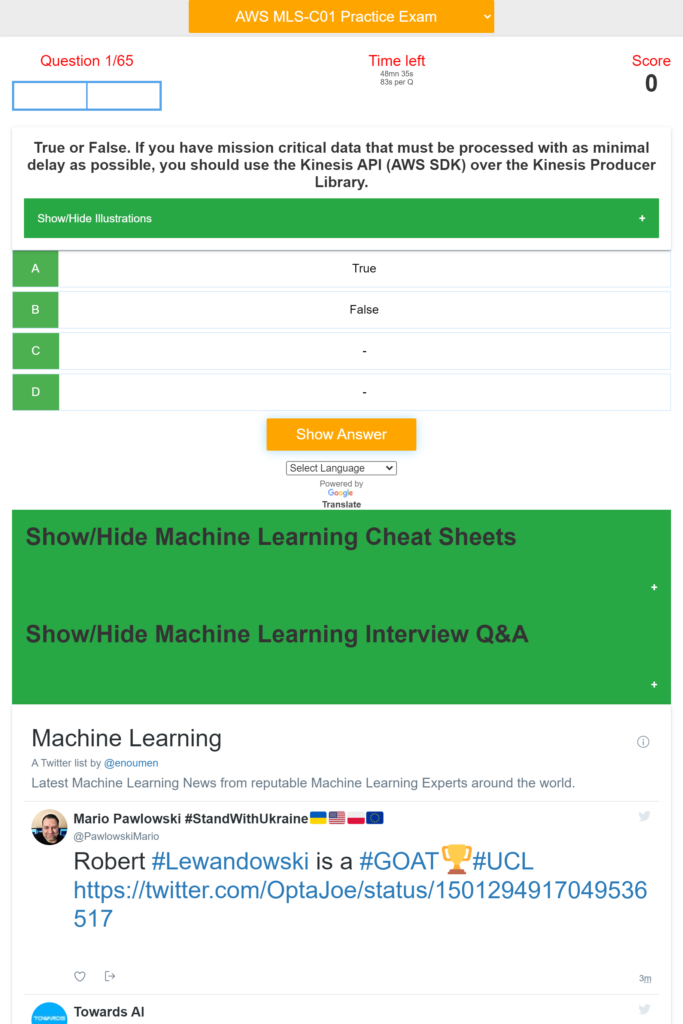
Download AWS machine Learning Specialty Exam Prep App on iOs
Download AWS Machine Learning Specialty Exam Prep App on Android/Web/Amazon
- Amazon SageMaker inference launches faster auto scaling for generative AI modelsby James Park (AWS Machine Learning Blog) on July 25, 2024 at 9:13 pm
Today, we are excited to announce a new capability in Amazon SageMaker inference that can help you reduce the time it takes for your generative artificial intelligence (AI) models to scale automatically. You can now use sub-minute metrics and significantly reduce overall scaling latency for generative AI models. With this enhancement, you can improve the
- Find answers accurately and quickly using Amazon Q Business with the SharePoint Online connectorby Vijai Gandikota (AWS Machine Learning Blog) on July 25, 2024 at 5:53 pm
Amazon Q Business is a fully managed, generative artificial intelligence (AI)-powered assistant that helps enterprises unlock the value of their data and knowledge. With Amazon Q, you can quickly find answers to questions, generate summaries and content, and complete tasks by using the information and expertise stored across your company’s various data sources and enterprise
- Evaluate conversational AI agents with Amazon Bedrockby Sharon Li (AWS Machine Learning Blog) on July 25, 2024 at 5:47 pm
As conversational artificial intelligence (AI) agents gain traction across industries, providing reliability and consistency is crucial for delivering seamless and trustworthy user experiences. However, the dynamic and conversational nature of these interactions makes traditional testing and evaluation methods challenging. Conversational AI agents also encompass multiple layers, from Retrieval Augmented Generation (RAG) to function-calling mechanisms that
- Node problem detection and recovery for AWS Neuron nodes within Amazon EKS clustersby Darren Lin (AWS Machine Learning Blog) on July 25, 2024 at 5:39 pm
In the post, we introduce the AWS Neuron node problem detector and recovery DaemonSet for AWS Trainium and AWS Inferentia on Amazon Elastic Kubernetes Service (Amazon EKS). This component can quickly detect rare occurrences of issues when Neuron devices fail by tailing monitoring logs. It marks the worker nodes in a defective Neuron device as unhealthy, and promptly replaces them with new worker nodes. By accelerating the speed of issue detection and remediation, it increases the reliability of your ML training and reduces the wasted time and cost due to hardware failure.
- Mistral Large 2 is now available in Amazon Bedrockby Niithiyn Vijeaswaran (AWS Machine Learning Blog) on July 24, 2024 at 8:14 pm
Mistral AI’s Mistral Large 2 (24.07) foundation model (FM) is now generally available in Amazon Bedrock. Mistral Large 2 is the newest version of Mistral Large, and according to Mistral AI offers significant improvements across multilingual capabilities, math, reasoning, coding, and much more. In this post, we discuss the benefits and capabilities of this new
- LLM experimentation at scale using Amazon SageMaker Pipelines and MLflowby Jagdeep Singh Soni (AWS Machine Learning Blog) on July 24, 2024 at 7:01 pm
Large language models (LLMs) have achieved remarkable success in various natural language processing (NLP) tasks, but they may not always generalize well to specific domains or tasks. You may need to customize an LLM to adapt to your unique use case, improving its performance on your specific dataset or task. You can customize the model
- Discover insights from Amazon S3 with Amazon Q S3 connector by Kruthi Jayasimha Rao (AWS Machine Learning Blog) on July 24, 2024 at 6:53 pm
Amazon Q is a fully managed, generative artificial intelligence (AI) powered assistant that you can configure to answer questions, provide summaries, generate content, gain insights, and complete tasks based on data in your enterprise. The enterprise data required for these generative-AI powered assistants can reside in varied repositories across your organization. One common repository to
- Boosting Salesforce Einstein’s code generating model performance with Amazon SageMakerby Pawan Agarwal (AWS Machine Learning Blog) on July 24, 2024 at 4:52 pm
This post is a joint collaboration between Salesforce and AWS and is being cross-published on both the Salesforce Engineering Blog and the AWS Machine Learning Blog. Salesforce, Inc. is an American cloud-based software company headquartered in San Francisco, California. It provides customer relationship management (CRM) software and applications focused on sales, customer service, marketing automation,
- Detect and protect sensitive data with Amazon Lex and Amazon CloudWatch Logsby Rashmica Gopinath (AWS Machine Learning Blog) on July 23, 2024 at 8:01 pm
In today’s digital landscape, the protection of personally identifiable information (PII) is not just a regulatory requirement, but a cornerstone of consumer trust and business integrity. Organizations use advanced natural language detection services like Amazon Lex for building conversational interfaces and Amazon CloudWatch for monitoring and analyzing operational data. One risk many organizations face is
- AWS AI chips deliver high performance and low cost for Llama 3.1 models on AWSby John Gray (AWS Machine Learning Blog) on July 23, 2024 at 4:18 pm
Today, we are excited to announce AWS Trainium and AWS Inferentia support for fine-tuning and inference of the Llama 3.1 models. The Llama 3.1 family of multilingual large language models (LLMs) is a collection of pre-trained and instruction tuned generative models in 8B, 70B, and 405B sizes. In a previous post, we covered how to deploy Llama 3 models on AWS Trainium and Inferentia based instances in Amazon SageMaker JumpStart. In this post, we outline how to get started with fine-tuning and deploying the Llama 3.1 family of models on AWS AI chips, to realize their price-performance benefits.
- Use Llama 3.1 405B for synthetic data generation and distillation to fine-tune smaller modelsby Sebastian Bustillo (AWS Machine Learning Blog) on July 23, 2024 at 4:18 pm
Today, we are excited to announce the availability of the Llama 3.1 405B model on Amazon SageMaker JumpStart, and Amazon Bedrock in preview. The Llama 3.1 models are a collection of state-of-the-art pre-trained and instruct fine-tuned generative artificial intelligence (AI) models in 8B, 70B, and 405B sizes. Amazon SageMaker JumpStart is a machine learning (ML) hub that provides access to algorithms, models, and ML solutions so you can quickly get started with ML. Amazon Bedrock offers a straightforward way to build and scale generative AI applications with Meta Llama models, using a single API.
- Llama 3.1 models are now available in Amazon SageMaker JumpStartby Saurabh Trikande (AWS Machine Learning Blog) on July 23, 2024 at 4:16 pm
Today, we are excited to announce that the state-of-the-art Llama 3.1 collection of multilingual large language models (LLMs), which includes pre-trained and instruction tuned generative AI models in 8B, 70B, and 405B sizes, is available through Amazon SageMaker JumpStart to deploy for inference. Llama is a publicly accessible LLM designed for developers, researchers, and businesses to build, experiment, and responsibly scale their generative artificial intelligence (AI) ideas. In this post, we walk through how to discover and deploy Llama 3.1 models using SageMaker JumpStart.
- Intelligent document processing using Amazon Bedrock and Anthropic Claudeby Govind Palanisamy (AWS Machine Learning Blog) on July 18, 2024 at 6:21 pm
In this post, we show how to develop an IDP solution using Anthropic Claude 3 Sonnet on Amazon Bedrock. We demonstrate how to extract data from a scanned document and insert it into a database.
- Metadata filtering for tabular data with Knowledge Bases for Amazon Bedrockby Tanay Chowdhury (AWS Machine Learning Blog) on July 18, 2024 at 6:19 pm
Amazon Bedrock is a fully managed service that offers a choice of high-performing foundation models (FMs) from leading artificial intelligence (AI) companies like AI21 Labs, Anthropic, Cohere, Meta, Mistral AI, Stability AI, and Amazon through a single API. To equip FMs with up-to-date and proprietary information, organizations use Retrieval Augmented Generation (RAG), a technique that
- Secure AccountantAI Chatbot: Lili’s journey with Amazon Bedrockby Doron Bleiberg (AWS Machine Learning Blog) on July 18, 2024 at 4:20 pm
This post was written in collaboration with Liran Zelkha and Eyal Solnik from Lili. Small business proprietors tend to prioritize the operational aspects of their enterprises over administrative tasks, such as maintaining financial records and accounting. While hiring a professional accountant can provide valuable guidance and expertise, it can be cost-prohibitive for many small businesses.
- How Mend.io unlocked hidden patterns in CVE data with Anthropic Claude on Amazon Bedrockby Hemmy Yona (AWS Machine Learning Blog) on July 18, 2024 at 4:14 pm
This post is co-written with Maciej Mensfeld from Mend.io. In the ever-evolving landscape of cybersecurity, the ability to effectively analyze and categorize Common Vulnerabilities and Exposures (CVEs) is crucial. This post explores how Mend.io, a cybersecurity firm, used Anthropic Claude on Amazon Bedrock to classify and identify CVEs containing specific attack requirements details. By using
- How Deloitte Italy built a digital payments fraud detection solution using quantum machine learning and Amazon Braketby Federica Marini (AWS Machine Learning Blog) on July 17, 2024 at 4:58 pm
As digital commerce expands, fraud detection has become critical in protecting businesses and consumers engaging in online transactions. Implementing machine learning (ML) algorithms enables real-time analysis of high-volume transactional data to rapidly identify fraudulent activity. This advanced capability helps mitigate financial risks and safeguard customer privacy within expanding digital markets. Deloitte is a strategic global
- Amazon SageMaker unveils the Cohere Command R fine-tuning modelby Shashi Raina (AWS Machine Learning Blog) on July 17, 2024 at 4:49 pm
AWS announced the availability of the Cohere Command R fine-tuning model on Amazon SageMaker. This latest addition to the SageMaker suite of machine learning (ML) capabilities empowers enterprises to harness the power of large language models (LLMs) and unlock their full potential for a wide range of applications. Cohere Command R is a scalable, frontier
- Derive meaningful and actionable operational insights from AWS Using Amazon Q Businessby Chitresh Saxena (AWS Machine Learning Blog) on July 17, 2024 at 4:36 pm
As a customer, you rely on Amazon Web Services (AWS) expertise to be available and understand your specific environment and operations. Today, you might implement manual processes to summarize lessons learned, obtain recommendations, or expedite the resolution of an incident. This can be time consuming, inconsistent, and not readily accessible. This post shows how to
- Accelerate your generative AI distributed training workloads with the NVIDIA NeMo Framework on Amazon EKSby Ankur Srivastava (AWS Machine Learning Blog) on July 16, 2024 at 7:56 pm
In today’s rapidly evolving landscape of artificial intelligence (AI), training large language models (LLMs) poses significant challenges. These models often require enormous computational resources and sophisticated infrastructure to handle the vast amounts of data and complex algorithms involved. Without a structured framework, the process can become prohibitively time-consuming, costly, and complex. Enterprises struggle with managing
Download AWS machine Learning Specialty Exam Prep App on iOs
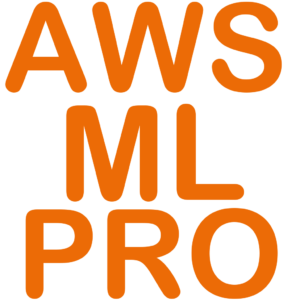
Download AWS Machine Learning Specialty Exam Prep App on Android/Web/Amazon
A Twitter List by enoumenDownload AWS machine Learning Specialty Exam Prep App on iOs
Download AWS Machine Learning Specialty Exam Prep App on Android/Web/Amazon