You can translate the content of this page by selecting a language in the select box.
The AWS Certified Machine Learning Specialty validates expertise in building, training, tuning, and deploying machine learning (ML) models on AWS.
Use this App to learn about Machine Learning on AWS and prepare for the AWS Machine Learning Specialty Certification MLS-C01.
Download AWS machine Learning Specialty Exam Prep App on iOs
Download AWS Machine Learning Specialty Exam Prep App on Android/Web/Amazon
[appbox appstore 1611045854-iphone screenshots]
[appbox microsoftstore 9n8rl80hvm4t-mobile screenshots]
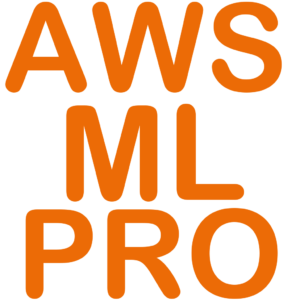
Download AWS machine Learning Specialty Exam Prep App on iOs
Download AWS Machine Learning Specialty Exam Prep App on Android/Web/Amazon
The App provides hundreds of quizzes and practice exam about:
– Machine Learning Operation on AWS
– Modelling
– Data Engineering
– Computer Vision,
– Exploratory Data Analysis,
– ML implementation & Operations
– Machine Learning Basics Questions and Answers
– Machine Learning Advanced Questions and Answers
– Scorecard
– Countdown timer
– Machine Learning Cheat Sheets
– Machine Learning Interview Questions and Answers
– Machine Learning Latest News
The App covers Machine Learning Basics and Advanced topics including: NLP, Computer Vision, Python, linear regression, logistic regression, Sampling, dataset, statistical interaction, selection bias, non-Gaussian distribution, bias-variance trade-off, Normal Distribution, correlation and covariance, Point Estimates and Confidence Interval, A/B Testing, p-value, statistical power of sensitivity, over-fitting and under-fitting, regularization, Law of Large Numbers, Confounding Variables, Survivorship Bias, univariate, bivariate and multivariate, Resampling, ROC curve, TF/IDF vectorization, Cluster Sampling, etc.
Domain 1: Data Engineering
Create data repositories for machine learning.
Identify data sources (e.g., content and location, primary sources such as user data)
Determine storage mediums (e.g., DB, Data Lake, S3, EFS, EBS)
Identify and implement a data ingestion solution.
Data job styles/types (batch load, streaming)
Data ingestion pipelines (Batch-based ML workloads and streaming-based ML workloads), etc.
Domain 2: Exploratory Data Analysis
Sanitize and prepare data for modeling.
Perform feature engineering.
Analyze and visualize data for machine learning.
Domain 3: Modeling
Frame business problems as machine learning problems.
Select the appropriate model(s) for a given machine learning problem.
Train machine learning models.
Perform hyperparameter optimization.
Evaluate machine learning models.
Domain 4: Machine Learning Implementation and Operations
Build machine learning solutions for performance, availability, scalability, resiliency, and fault
tolerance.
Recommend and implement the appropriate machine learning services and features for a given
problem.
Apply basic AWS security practices to machine learning solutions.
Deploy and operationalize machine learning solutions.
Machine Learning Services covered:
Amazon Comprehend
AWS Deep Learning AMIs (DLAMI)
AWS DeepLens
Amazon Forecast
Amazon Fraud Detector
Amazon Lex
Amazon Polly
Amazon Rekognition
Amazon SageMaker
Amazon Textract
Amazon Transcribe
Amazon Translate
Other Services and topics covered are:
Ingestion/Collection
Processing/ETL
Data analysis/visualization
Model training
Model deployment/inference
Operational
AWS ML application services
Language relevant to ML (for example, Python, Java, Scala, R, SQL)
Notebooks and integrated development environments (IDEs),
S3, SageMaker, Kinesis, Lake Formation, Athena, Kibana, Redshift, Textract, EMR, Glue, SageMaker, CSV, JSON, IMG, parquet or databases, Amazon Athena
Amazon EC2, Amazon Elastic Container Registry (Amazon ECR), Amazon Elastic Container Service, Amazon Elastic Kubernetes Service , Amazon Redshift
Important: To succeed with the real exam, do not memorize the answers in this app. It is very important that you understand why a question is right or wrong and the concepts behind it by carefully reading the reference documents in the answers.
Note and disclaimer: We are not affiliated with Microsoft or Azure or Google or Amazon. The questions are put together based on the certification study guide and materials available online. The questions in this app should help you pass the exam but it is not guaranteed. We are not responsible for any exam you did not pass.
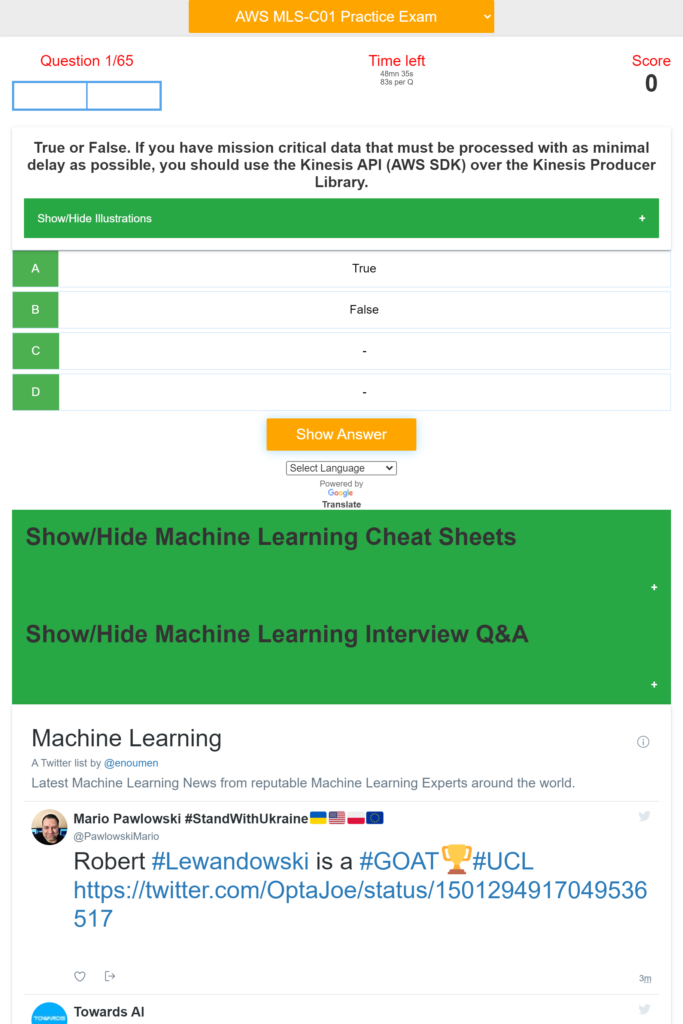
Download AWS machine Learning Specialty Exam Prep App on iOs
Download AWS Machine Learning Specialty Exam Prep App on Android/Web/Amazon
- [R] Collaboration. Veterinary surgeon Seeking ML Experts for Feline and Canine Ophthalmic Projectby /u/thegovet (Machine Learning) on July 26, 2024 at 5:52 pm
I'm an Advanced Practitioner in Veterinary Ophthalmology ophthalmology. I'm looking to build a machine learning model to detect eye pathologies in cats and dogs using image analysis. I have a dataset of a few dozen images but I'm well connected in the veterinary ophthalmology world and can get more. It would include both healthy and diseased eyes. I'm proficient in Python but need expertise in image processing, deep learning, and model development. I'm open to collaborating with anyone interested in this project. We can work together to build a valuable tool for veterinarians worldwide. If you're passionate about AI and animal health, please reach out! Let's discuss this project further. AI #machinelearning #veterinary #ophthalmology #collaboration #opendata submitted by /u/thegovet [link] [comments]
- [D] Every annotator has a guidebook, but the reviewers don'tby /u/Spico197 (Machine Learning) on July 26, 2024 at 1:20 pm
I submitted to the ACL rolling review in June and found the reviewers' evaluation scores very subjective. Although the ACL committee has an instruction on some basic reviewing guidelines, there lacks of a preliminary test for the reviewers to explicitly show the evaluation standards. Maybe we should provide some paper-score examples to prompt the reviewers for more objective reviews? Or build a test before they make reviews to make sure they fully understand the meaning of soundness and overall assessment, rather than giving some random scores based on their personal interests. submitted by /u/Spico197 [link] [comments]
- [D] How OpenAI JSON mode implemented?by /u/Financial_Air5256 (Machine Learning) on July 26, 2024 at 11:15 am
I assume that all the training data is in JSON format, but higher temperature or other randomness during generation doesn’t guarantee that the outputs will always be in JSON. What other methods do you think could ensure that the outputs are consistently in JSON? Perhaps some rule-based methods during decoding could help? submitted by /u/Financial_Air5256 [link] [comments]
- [D] Do only some hardware support int4 quantization? If so why?by /u/Abs0lute_Jeer0 (Machine Learning) on July 26, 2024 at 7:56 am
I tried quantizing my finetuned mT5 model using the optimum’s openvino wrapper to int8 and int4. There was very little difference in the inference time, close to 5%. This makes me wonder if it’s an issue with hardware. I’m using intel sapphire rapids and it has an avx512_vnni instruction set. How did I figure out if it supports int4? And why and why not? submitted by /u/Abs0lute_Jeer0 [link] [comments]
- [D] Normalization in transformersby /u/lostn4d (Machine Learning) on July 26, 2024 at 7:44 am
After the first theoretical issue with my transformer, I now see another. The original paper uses normalization after residual addition (Post-LN), which led to training difficulties and later got replaced by normalization at the beginning of each attention or mlp block/branch (Pre-LN). This is known to work better in practice (trainable without warmup, restores highway effect), but it still doesn't seem completely ok theoretically. First consider things without normalization. Assuming attention and mlp blocks are properly set up and mostly keep norms, each residual addition would sum two similar norm signals, potentially scaling up by something like 1.4 (depending on correlation, but it starts at sqrt(2) after random init). So the norms after the blocks could look like this: [1(main)+1(residual)=1.4] -> [1.4+1.4=2] -> [2+2=2.8] etc. This would cause various problems (like changing the softmax temp in later attention blocks), so adjustment is needed. Pre-LN ensures each block works on normalized values (thus with constant - if slightly arbitrary - softmax temperature). But since it doesn't affect the norm of the main signal (as forwarded by the skip connection) but only the residual, the norms can still grow, albeit slower. The expectation is now roughly: [1+1=1.4] -> [1.4+1=1.7] -> [1.7+1=2] -> [2+1=2.2] etc - with a final normalization correcting the signal near output (Pre-LN paper). One possible issue with this is that later attention blocks may have reduced effect, as they add unit norm residuals to a potentially larger and larger main signal. What is the usual take on this problem? Can it be ignored in practice? Does Pre-LN work acceptably despite it, even for deep models (where the main norm discrepancy can grow larger)? There are lots of alternative normalization papers, but what is the practical consensus? Btw attention is extremely norm-sensitive (or, equivalently, the hidden temperature of softmax is critical). This is a sharp contrast to fc or convolution which are mostly scale-oblivious. For anybody interested: consider what happens when most raw attention dot products come out 0 (= query and key is orthogonal, no info from this context slot) with only one slot giving 1 (= positive affinity, after downscaled by sqrt(qk_siz) ). I for one got surprised by this during debug. submitted by /u/lostn4d [link] [comments]
- [R] How do you search for implementations of Mixture of Expert models that can be trained locally in a laptop or desktop without ultra-high end GPUs?by /u/Furiousguy79 (Machine Learning) on July 25, 2024 at 11:12 pm
Hi, I am a 2nd year PhD student in CS. My supervisor just got this idea about MoEs and fairness and asked me to implement it ( work on a toy classification problem on tabular data and NOT language data). However as it is not their area of expertise, they did not give any guidelines on how to approach it. My main question is: How do I search for or proceed with implementing a mixture of expert models? The ones that I find are for chatting and such but I mainly work with tabular EHR data. This is my first foray into this area (LLMs and MoEs) and I am kind of lost with all these Mixtral, openMoE, etc. As we do not have access to Google Collab or have powerful GPUs I have to rely on local training (My lab PC has 2080ti and my laptop has 4070). Any guideline or starting point on how to proceed would be greatly appreciated. submitted by /u/Furiousguy79 [link] [comments]
- Amazon SageMaker inference launches faster auto scaling for generative AI modelsby James Park (AWS Machine Learning Blog) on July 25, 2024 at 9:13 pm
Today, we are excited to announce a new capability in Amazon SageMaker inference that can help you reduce the time it takes for your generative artificial intelligence (AI) models to scale automatically. You can now use sub-minute metrics and significantly reduce overall scaling latency for generative AI models. With this enhancement, you can improve the
- [P] How to make "Out-of-sample" Predictionsby /u/Individual_Ad_1214 (Machine Learning) on July 25, 2024 at 7:47 pm
My data is a bit complicated to describe so I'm going try to describe something analogous. Each example is randomly generated, but you can group them based on a specific but latent (by latent I mean this isn't added into the features used to develop a model, but I have access to it) feature (in this example we'll call this number of bedrooms). Feature x1 Feature x2 Feature x3 ... Output (Rent) Row 1 Row 2 Row 3 Row 4 Row 5 Row 6 Row 7 2 Row 8 1 Row 9 0 So I can group Row 1, Row 2, and Row 3 based on a latent feature called number of bedrooms (which in this case is 0 bedroom). Similarly, Row 4, Row 5, & Row 6 have 2 Bedrooms, and Row 7, Row 8, & Row 9 have 4 Bedrooms. Furthermore, these groups also have an optimum price which is used to create output classes (output here is Rent; increase, keep constant, or decrease). So say the optimum price for the 4 bedrooms group is $3mil, and row 7 has a price of $4mil (=> 3 - 4 = -1 mil, i.e a -ve value so convert this to class 2, or above optimum or increase rent), row 8 has a price of $3mil (=> 3 - 3 = 0, convert this to class 1, or at optimum), and row 9 has a price of $2mil (3 - 2 = 1, i.e +ve value, so convert this to class 0, or below optimum, or decrease rent). I use this method to create an output class for each example in the dataset (essentially, if example x has y number of bedrooms, I get the known optimum price for that number of bedrooms and I subtract the example's price from the optimum price). Say I have 10 features (e.g. square footage, number of bathrooms, parking spaces etc.) in the dataset, these 10 features provide the model with enough information to figure out the "number of bedrooms". So when I am evaluating the model, feature x1 feature x2 feature x3 ... Row 10 e.g. I pass into the model a test example (Row 10) which I know has 4 bedrooms and is priced at $6mil, the model can accurately predict class 2 (i.e increase rent) for this example. Because the model was developed using data with a representative number of bedrooms in my dataset. Features.... Output (Rent) Row 1 0 Row 2 0 Row 3 0 However, my problem arises at examples with a low number of bedrooms (i.e. 0 bedrooms). The input features doesn't have enough information to determine the number of bedrooms for examples with a low number of bedrooms (which is fine because we assume that within this group, we will always decrease the rent, so we set the optimum price to say $2000. So row 1 price could be $8000, (8000 - 2000 = 6000, +ve value thus convert to class 0 or below optimum/decrease rent). And within this group we rely on the class balance to help the model learn to make predictions because the proportion is heavily skewed towards class 0 (say 95% = class 0 or decrease rent, and 5 % = class 1 or class 2). We do this based the domain knowledge of the data (so in this case, we would always decrease the rent because no one wants to live in a house with 0 bedrooms). MAIN QUESTION: We now want to predict (or undertake inference) for examples with number of bedrooms in between 0 bedrooms and 2 bedrooms (e.g 1 bedroom NOTE: our training data has no example with 1 bedroom). What I notice is that the model's predictions on examples with 1 bedroom act as if these examples had 0 bedrooms and it mostly predicts class 0. My question is, apart from specifically including examples with 1 bedroom in my input data, is there any other way (more statistics or ML related way) for me to improve the ability of my model to generalise on unseen data? submitted by /u/Individual_Ad_1214 [link] [comments]
- [R] EMNLP Paper review scoresby /u/Immediate-Hour-8466 (Machine Learning) on July 25, 2024 at 7:06 pm
EMNLP paper review scores Overall assessment for my paper is 2, 2.5 and 3. Is there any chance that it may still be selected? The confidence is 2, 2.5 and 3. The soundness is 2, 2.5, 3.5. I am not sure how soundness and confidence may affect my paper's selection. Pls explain how this works. Which metrics should I consider important. Thank you! submitted by /u/Immediate-Hour-8466 [link] [comments]
- [N] OpenAI announces SearchGPTby /u/we_are_mammals (Machine Learning) on July 25, 2024 at 6:41 pm
https://openai.com/index/searchgpt-prototype/ We’re testing SearchGPT, a temporary prototype of new AI search features that give you fast and timely answers with clear and relevant sources. submitted by /u/we_are_mammals [link] [comments]
- Find answers accurately and quickly using Amazon Q Business with the SharePoint Online connectorby Vijai Gandikota (AWS Machine Learning Blog) on July 25, 2024 at 5:53 pm
Amazon Q Business is a fully managed, generative artificial intelligence (AI)-powered assistant that helps enterprises unlock the value of their data and knowledge. With Amazon Q, you can quickly find answers to questions, generate summaries and content, and complete tasks by using the information and expertise stored across your company’s various data sources and enterprise
- Evaluate conversational AI agents with Amazon Bedrockby Sharon Li (AWS Machine Learning Blog) on July 25, 2024 at 5:47 pm
As conversational artificial intelligence (AI) agents gain traction across industries, providing reliability and consistency is crucial for delivering seamless and trustworthy user experiences. However, the dynamic and conversational nature of these interactions makes traditional testing and evaluation methods challenging. Conversational AI agents also encompass multiple layers, from Retrieval Augmented Generation (RAG) to function-calling mechanisms that
- Node problem detection and recovery for AWS Neuron nodes within Amazon EKS clustersby Darren Lin (AWS Machine Learning Blog) on July 25, 2024 at 5:39 pm
In the post, we introduce the AWS Neuron node problem detector and recovery DaemonSet for AWS Trainium and AWS Inferentia on Amazon Elastic Kubernetes Service (Amazon EKS). This component can quickly detect rare occurrences of issues when Neuron devices fail by tailing monitoring logs. It marks the worker nodes in a defective Neuron device as unhealthy, and promptly replaces them with new worker nodes. By accelerating the speed of issue detection and remediation, it increases the reliability of your ML training and reduces the wasted time and cost due to hardware failure.
- [P] Local Llama 3.1 and Marqo Retrieval Augmented Generationby /u/elliesleight (Machine Learning) on July 25, 2024 at 4:45 pm
I built a simple starter demo of a Knowledge Question and Answering System using Llama 3.1 (8B GGUF) and Marqo. Feel free to experiment and build on top of this yourselves! GitHub: https://github.com/ellie-sleightholm/marqo-llama3_1 submitted by /u/elliesleight [link] [comments]
- [N] AI achieves silver-medal standard solving International Mathematical Olympiad problemsby /u/we_are_mammals (Machine Learning) on July 25, 2024 at 4:16 pm
https://deepmind.google/discover/blog/ai-solves-imo-problems-at-silver-medal-level/ They solved 4 of the 6 IMO problems (although it took days to solve some of them). This would have gotten them a score of 28/42, just one point below the gold-medal level. submitted by /u/we_are_mammals [link] [comments]
- [R] Explainability of HuggingFace Models (LLMs) for Text Summarization/Generation Tasksby /u/PhoenixHeadshot25 (Machine Learning) on July 25, 2024 at 3:19 pm
Hi community, I am exploring the Responsible AI domain where I have started reading about methods and tools to make Deep Learning Models explainable. I have already used SHAP and LIMe for ML model explainability. However, I am unsure about their use in explaining LLMs. I know that these methods are model agnostic but can we use these methods for Text Generation or Summarization tasks? I got reference docs from Shap explaining GPT2 for text generation tasks, but I am unsure about using it for other newer LLMs. Additionally, I would like to know, are there any better ways for Explainable AI for LLMs? submitted by /u/PhoenixHeadshot25 [link] [comments]
- [D] High-Dimensional Probabilistic Modelsby /u/smorad (Machine Learning) on July 25, 2024 at 2:58 pm
What is the standard way to model high-dimensional stochastic processes today? I have some process defined over images x, and I would like to compute P(x' | x, z) for all x'. I know there are Normalizing Flows, Gaussian Processes, etc, but I do not know which to get started with. I specifically want to compute the probabilities, not just sample some x' ~ P(x, z). submitted by /u/smorad [link] [comments]
- [R] Shared Imagination: LLMs Hallucinate Alikeby /u/zyl1024 (Machine Learning) on July 25, 2024 at 1:49 pm
Happy to share our recent paper, where we demonstrate that LLMs exhibit surprising agreement on purely imaginary and hallucinated contents -- what we call a "shared imagination space". To arrive at this conclusion, we ask LLMs to generate questions on hypothetical contents (e.g., a made-up concept in physics) and then find that they can answer each other's (unanswerable and nonsensical) questions with much higher accuracy than random chance. From this, we investigate in multiple directions on its emergence, generality and possible reasons, and given such consistent hallucination and imagination behavior across modern LLMs, discuss implications to hallucination detection and computational creativity. Link to the paper: https://arxiv.org/abs/2407.16604 Link to the tweet with result summary and highlight: https://x.com/YilunZhou/status/1816371178501476473 Please feel free to ask any questions! The main experiment setup and finding. submitted by /u/zyl1024 [link] [comments]
- [R] Paper NAACL 2024: "Reliability Estimation of News Media Sources: Birds of a Feather Flock Together"by /u/sergbur (Machine Learning) on July 25, 2024 at 9:10 am
For people working on information verification in general, for instance, working on fact checking, fake news detection or even using RAG from news articles this paper may be useful. Authors use different reinforcement learning techniques to estimate reliability values of news media outlets based on how they interact on the web. The method is easy to scale since the source code is available to build larger hyperlink-based interaction graphs from Common Crawl News. Authors also released the computed values and dataset with news media reliability annotation: Github repo: https://github.com/idiap/News-Media-Reliability Paper: https://aclanthology.org/2024.naacl-long.383/ Live Demo Example: https://lab.idiap.ch/criteria/ In the demo, the retrieved news articles will be order not only by the match to the query but also by the estimated reliability for each sources (URL domains are color coded from green to red, for instance, scrolling down will show results coming from less reliable sources marked with red-ish colors). Alternatively, if a news URL or a news outlet domain (e.g. apnews.com) is given as a query, information about the estimated values are detailed (e.g. showing the neighboring sources interacting with the media, etc.) Have a nice day, everyone! 🙂 submitted by /u/sergbur [link] [comments]
- [D] ACL ARR June (EMNLP) Review Discussionby /u/always_been_a_toy (Machine Learning) on July 25, 2024 at 4:45 am
Too anxious about reviews as they didn’t arrive yet! Wanted to share with the community and see the reactions to the reviews! Rant and stuff! Be polite in comments. submitted by /u/always_been_a_toy [link] [comments]
- "[Discussion]" Where do you get your updates on latest research in video generation and computer vision?by /u/Sobieski526 (Machine Learning) on July 24, 2024 at 9:20 pm
As the title says, looking for some tips on how you keep track of the latest research in video generation and CV. I have been reading through https://cvpr.thecvf.com/ and it's a great source, are there any simiar ones? submitted by /u/Sobieski526 [link] [comments]
- [R] Pre-prompting your LLM increases performanceby /u/CalendarVarious3992 (Machine Learning) on July 24, 2024 at 8:33 pm
Research done at UoW shows that pre-prompting your LLM, or providing context prior to asking your question leads to better results. Even when the context is self generated. https://arxiv.org/pdf/2110.08387 For example asking, "What should I do while in Rome?" is less effective than a series of prompts, "What are the top restaraunts in Rome?" "What are the top sight seeing locations in Rome?" "Best things to do in Rome" "What should I do in Rome?" I always figured this was the case from anecdotal evidence but good to see people who are way starter than me explain it in this paper. And while chain prompting is a little more time consuming there's chrome extensions like ChatGPT Queue that ease up the process. Are their any other "hacks" to squeeze out better performance ? submitted by /u/CalendarVarious3992 [link] [comments]
- [R] Segment Anything Repository Archived - Why?by /u/Ben-L-921 (Machine Learning) on July 24, 2024 at 8:23 pm
Hello ML subreddit, I was recently made aware of the fact that the segment anything repository got made into a public archive less than a month ago (July 1st, 2024). I was not able to find any information pertaining to why this was the case, however. I know there have been a lot of derivatives of segment anything in development, but I don't know why this would have warranted a public archive. Does anyone know why this happened and where we might be able to redirect questions/issues for the work? submitted by /u/Ben-L-921 [link] [comments]
- Mistral Large 2 is now available in Amazon Bedrockby Niithiyn Vijeaswaran (AWS Machine Learning Blog) on July 24, 2024 at 8:14 pm
Mistral AI’s Mistral Large 2 (24.07) foundation model (FM) is now generally available in Amazon Bedrock. Mistral Large 2 is the newest version of Mistral Large, and according to Mistral AI offers significant improvements across multilingual capabilities, math, reasoning, coding, and much more. In this post, we discuss the benefits and capabilities of this new
- [N] Mistral releases a "Large Enough" modelby /u/we_are_mammals (Machine Learning) on July 24, 2024 at 7:04 pm
https://mistral.ai/news/mistral-large-2407/ 123B parameters On par with GPT-4o and Llama 3.1 405B, according to their benchmarks Mistral Research License allows usage and modification for research and non-commercial purposes submitted by /u/we_are_mammals [link] [comments]
- LLM experimentation at scale using Amazon SageMaker Pipelines and MLflowby Jagdeep Singh Soni (AWS Machine Learning Blog) on July 24, 2024 at 7:01 pm
Large language models (LLMs) have achieved remarkable success in various natural language processing (NLP) tasks, but they may not always generalize well to specific domains or tasks. You may need to customize an LLM to adapt to your unique use case, improving its performance on your specific dataset or task. You can customize the model
- Discover insights from Amazon S3 with Amazon Q S3 connector by Kruthi Jayasimha Rao (AWS Machine Learning Blog) on July 24, 2024 at 6:53 pm
Amazon Q is a fully managed, generative artificial intelligence (AI) powered assistant that you can configure to answer questions, provide summaries, generate content, gain insights, and complete tasks based on data in your enterprise. The enterprise data required for these generative-AI powered assistants can reside in varied repositories across your organization. One common repository to
- Boosting Salesforce Einstein’s code generating model performance with Amazon SageMakerby Pawan Agarwal (AWS Machine Learning Blog) on July 24, 2024 at 4:52 pm
This post is a joint collaboration between Salesforce and AWS and is being cross-published on both the Salesforce Engineering Blog and the AWS Machine Learning Blog. Salesforce, Inc. is an American cloud-based software company headquartered in San Francisco, California. It provides customer relationship management (CRM) software and applications focused on sales, customer service, marketing automation,
- [P] NCCLX mentioned in llama3 paperby /u/khidot (Machine Learning) on July 24, 2024 at 3:54 pm
The paper says `Our collective communication library for Llama 3 is based on a fork of Nvidia’s NCCL library, called NCCLX. NCCLX significantly improves the performance of NCCL, especially for higher latency networks`. Can anyone give more background? Any plans to release or upstream? Any more technical details? submitted by /u/khidot [link] [comments]
- [R] Scaling Diffusion Transformers to 16 Billion Parametersby /u/StartledWatermelon (Machine Learning) on July 24, 2024 at 3:12 pm
TL;DR Adding Mixture-of-Experts into a Diffusion Transformer gets you an efficient and powerful model. Paper: https://arxiv.org/pdf/2407.11633 Abstract: In this paper, we present DiT-MoE, a sparse version of the diffusion Transformer, that is scalable and competitive with dense networks while exhibiting highly optimized inference. The DiT-MoE includes two simple designs: shared expert routing and expert-level balance loss, thereby capturing common knowledge and reducing redundancy among the different routed experts. When applied to conditional image generation, a deep analysis of experts specialization gains some interesting observations: (i) Expert selection shows preference with spatial position and denoising time step, while insensitive with different class-conditional information; (ii) As the MoE layers go deeper, the selection of experts gradually shifts from specific spacial position to dispersion and balance. (iii) Expert specialization tends to be more concentrated at the early time step and then gradually uniform after half. We attribute it to the diffusion process that first models the low-frequency spatial information and then high-frequency complex information. Based on the above guidance, a series of DiT-MoE experimentally achieves performance on par with dense networks yet requires much less computational load during inference. More encouragingly, we demonstrate the potential of DiT-MoE with synthesized image data, scaling diffusion model at a 16.5B parameter that attains a new SoTA FID-50K score of 1.80 in 512×512 resolution settings. The project page: this https URL. Visual Abstract: https://preview.redd.it/cq6yoqoeched1.png?width=1135&format=png&auto=webp&s=1985119b5150c76bb9807f4df45d7bb44e02bd2a Visual Highlights: https://preview.redd.it/8xf8egk9dhed1.png?width=1109&format=png&auto=webp&s=6e25b12d9a89d78847945068469f83cb45ef1eab 1S, 2S and 4S in the middle panel refer to the number of shared experts MoE decreases training stability, but not catastrophically https://preview.redd.it/s6cchx2nehed1.png?width=983&format=png&auto=webp&s=c426ce2f1362bace2b4d3abef8d7e5607d0ff405 submitted by /u/StartledWatermelon [link] [comments]
- [N] ICML 2024 liveblogby /u/hcarlens (Machine Learning) on July 24, 2024 at 1:30 pm
I'm doing an ICML liveblog, for people who aren't attending or are attending virtually and want to get more of a feel of the conference. In the past I've found it's not easy to get a good feel for a conference just from the conference website and Twitter. I'm trying to cover as much as I can, but obviously there are lots of simultaneous sessions and only so many hours in the day! If there's anything you'd like me to cover, give me a shout. Liveblog is here: https://mlcontests.com/icml-2024/?ref=mlcr If you're there in-person, come say hi! The official ICML website is here: https://icml.cc/ submitted by /u/hcarlens [link] [comments]
- [R] Low rank field-weighted factorization machinesby /u/alexsht1 (Machine Learning) on July 24, 2024 at 9:40 am
Our paper 'Low Rank Field-Weighted Factorization Machines for Low Latency Item Recommendation', by Alex Shtoff, Michael Viderman, Naama Haramaty-Krasne, Oren Somekh, Ariel Raviv, and Tularam Ban, has been accepted to RecSys 2024. I believe it's of interest to the ML-driven recommender system community. I think it's especially interesting to researchers working on large scale systems operating under extreme time constraints, such as online advertising. TL;DR: We reduce the cost of inference of FwFM models with n features and nᵢ item features from O(n²) to O(c nᵢ), where c is a small constant. This is to facilitate much cheaper large scale real-time inference for item recommendation. Code and paper: GitHub link. Details FMs are widely used in online advertising because they strike a good balance between representation power, and blazing fast training and inference speed. It is is paramount for large scale recommendation under tight time constraints. The main trick devised by Rendle et. al is computing *pairwise* interactions of n features in O(n) time. Moreover, user / context features, which are the same when ranking multiple items for a given user, can be handled separately (see the image below). The computational cost of a single recommendation becomes O(nᵢ) per item, where nᵢ is the number of item features. Consequently, adding more user or context features is practically free. FM formula in linear time The more advanced variants, such as Field-Aware and Field-Weighted FMs do not enjoy this property and require O(n²) time. This poses a challenge to such systems, and requires carefully thinking weather an additional user or context feature is worth the cost at inference. Typically, aggressive pruning of the field interactions is employed to dramatically reduce the computational cost, at the expense of model accuracy. In this work we devise a reformulation of the Field-Weighted FM family using diagonal plus low-rank (DPLR) factorization of the field interaction matrix, that facilitates inference in O(c nᵢ) time per item, where c is a small constant that we control. As is the case with pruning, the price is a slight reduction in model accuracy. We show that with a comparable number of parameters, the DPLR variant outperforms pruning on real world datasets, while facilitating significantly faster inference speeds, and gaining back the ability to add user context items practically for free. Here is a short chart summarizing the results: Diagonal+LowRank (DPLR) inference time significantly outperforms pruned time, and decreases quickly as the portion of context features (out of 40 total features) is increased. Plotted for various ad auction sizes and model ranks. submitted by /u/alexsht1 [link] [comments]
- getting into Diffusion Models [D]by /u/Same_Half3758 (Machine Learning) on July 24, 2024 at 8:45 am
Hello, I have noticed several papers focusing on diffusion models at CVPR 2024, particularly those related to Point Clouds. As I am quite new to this concept, I was wondering what would be a good starting point to better understand it? to save time and energy I am also working on Point Clouds, BTW. thank you submitted by /u/Same_Half3758 [link] [comments]
- Detect and protect sensitive data with Amazon Lex and Amazon CloudWatch Logsby Rashmica Gopinath (AWS Machine Learning Blog) on July 23, 2024 at 8:01 pm
In today’s digital landscape, the protection of personally identifiable information (PII) is not just a regulatory requirement, but a cornerstone of consumer trust and business integrity. Organizations use advanced natural language detection services like Amazon Lex for building conversational interfaces and Amazon CloudWatch for monitoring and analyzing operational data. One risk many organizations face is
- AWS AI chips deliver high performance and low cost for Llama 3.1 models on AWSby John Gray (AWS Machine Learning Blog) on July 23, 2024 at 4:18 pm
Today, we are excited to announce AWS Trainium and AWS Inferentia support for fine-tuning and inference of the Llama 3.1 models. The Llama 3.1 family of multilingual large language models (LLMs) is a collection of pre-trained and instruction tuned generative models in 8B, 70B, and 405B sizes. In a previous post, we covered how to deploy Llama 3 models on AWS Trainium and Inferentia based instances in Amazon SageMaker JumpStart. In this post, we outline how to get started with fine-tuning and deploying the Llama 3.1 family of models on AWS AI chips, to realize their price-performance benefits.
- Use Llama 3.1 405B for synthetic data generation and distillation to fine-tune smaller modelsby Sebastian Bustillo (AWS Machine Learning Blog) on July 23, 2024 at 4:18 pm
Today, we are excited to announce the availability of the Llama 3.1 405B model on Amazon SageMaker JumpStart, and Amazon Bedrock in preview. The Llama 3.1 models are a collection of state-of-the-art pre-trained and instruct fine-tuned generative artificial intelligence (AI) models in 8B, 70B, and 405B sizes. Amazon SageMaker JumpStart is a machine learning (ML) hub that provides access to algorithms, models, and ML solutions so you can quickly get started with ML. Amazon Bedrock offers a straightforward way to build and scale generative AI applications with Meta Llama models, using a single API.
- Llama 3.1 models are now available in Amazon SageMaker JumpStartby Saurabh Trikande (AWS Machine Learning Blog) on July 23, 2024 at 4:16 pm
Today, we are excited to announce that the state-of-the-art Llama 3.1 collection of multilingual large language models (LLMs), which includes pre-trained and instruction tuned generative AI models in 8B, 70B, and 405B sizes, is available through Amazon SageMaker JumpStart to deploy for inference. Llama is a publicly accessible LLM designed for developers, researchers, and businesses to build, experiment, and responsibly scale their generative artificial intelligence (AI) ideas. In this post, we walk through how to discover and deploy Llama 3.1 models using SageMaker JumpStart.
- [D] Self-Promotion Threadby /u/AutoModerator (Machine Learning) on July 21, 2024 at 2:15 am
Please post your personal projects, startups, product placements, collaboration needs, blogs etc. Please mention the payment and pricing requirements for products and services. Please do not post link shorteners, link aggregator websites , or auto-subscribe links. Any abuse of trust will lead to bans. Encourage others who create new posts for questions to post here instead! Thread will stay alive until next one so keep posting after the date in the title. Meta: This is an experiment. If the community doesnt like this, we will cancel it. This is to encourage those in the community to promote their work by not spamming the main threads. submitted by /u/AutoModerator [link] [comments]
- Intelligent document processing using Amazon Bedrock and Anthropic Claudeby Govind Palanisamy (AWS Machine Learning Blog) on July 18, 2024 at 6:21 pm
In this post, we show how to develop an IDP solution using Anthropic Claude 3 Sonnet on Amazon Bedrock. We demonstrate how to extract data from a scanned document and insert it into a database.
- Metadata filtering for tabular data with Knowledge Bases for Amazon Bedrockby Tanay Chowdhury (AWS Machine Learning Blog) on July 18, 2024 at 6:19 pm
Amazon Bedrock is a fully managed service that offers a choice of high-performing foundation models (FMs) from leading artificial intelligence (AI) companies like AI21 Labs, Anthropic, Cohere, Meta, Mistral AI, Stability AI, and Amazon through a single API. To equip FMs with up-to-date and proprietary information, organizations use Retrieval Augmented Generation (RAG), a technique that
- Secure AccountantAI Chatbot: Lili’s journey with Amazon Bedrockby Doron Bleiberg (AWS Machine Learning Blog) on July 18, 2024 at 4:20 pm
This post was written in collaboration with Liran Zelkha and Eyal Solnik from Lili. Small business proprietors tend to prioritize the operational aspects of their enterprises over administrative tasks, such as maintaining financial records and accounting. While hiring a professional accountant can provide valuable guidance and expertise, it can be cost-prohibitive for many small businesses.
- How Mend.io unlocked hidden patterns in CVE data with Anthropic Claude on Amazon Bedrockby Hemmy Yona (AWS Machine Learning Blog) on July 18, 2024 at 4:14 pm
This post is co-written with Maciej Mensfeld from Mend.io. In the ever-evolving landscape of cybersecurity, the ability to effectively analyze and categorize Common Vulnerabilities and Exposures (CVEs) is crucial. This post explores how Mend.io, a cybersecurity firm, used Anthropic Claude on Amazon Bedrock to classify and identify CVEs containing specific attack requirements details. By using
- How Deloitte Italy built a digital payments fraud detection solution using quantum machine learning and Amazon Braketby Federica Marini (AWS Machine Learning Blog) on July 17, 2024 at 4:58 pm
As digital commerce expands, fraud detection has become critical in protecting businesses and consumers engaging in online transactions. Implementing machine learning (ML) algorithms enables real-time analysis of high-volume transactional data to rapidly identify fraudulent activity. This advanced capability helps mitigate financial risks and safeguard customer privacy within expanding digital markets. Deloitte is a strategic global
- Amazon SageMaker unveils the Cohere Command R fine-tuning modelby Shashi Raina (AWS Machine Learning Blog) on July 17, 2024 at 4:49 pm
AWS announced the availability of the Cohere Command R fine-tuning model on Amazon SageMaker. This latest addition to the SageMaker suite of machine learning (ML) capabilities empowers enterprises to harness the power of large language models (LLMs) and unlock their full potential for a wide range of applications. Cohere Command R is a scalable, frontier
- Derive meaningful and actionable operational insights from AWS Using Amazon Q Businessby Chitresh Saxena (AWS Machine Learning Blog) on July 17, 2024 at 4:36 pm
As a customer, you rely on Amazon Web Services (AWS) expertise to be available and understand your specific environment and operations. Today, you might implement manual processes to summarize lessons learned, obtain recommendations, or expedite the resolution of an incident. This can be time consuming, inconsistent, and not readily accessible. This post shows how to
- Accelerate your generative AI distributed training workloads with the NVIDIA NeMo Framework on Amazon EKSby Ankur Srivastava (AWS Machine Learning Blog) on July 16, 2024 at 7:56 pm
In today’s rapidly evolving landscape of artificial intelligence (AI), training large language models (LLMs) poses significant challenges. These models often require enormous computational resources and sophisticated infrastructure to handle the vast amounts of data and complex algorithms involved. Without a structured framework, the process can become prohibitively time-consuming, costly, and complex. Enterprises struggle with managing
Download AWS machine Learning Specialty Exam Prep App on iOs
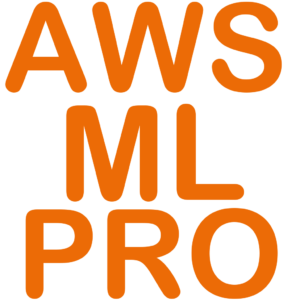
Download AWS Machine Learning Specialty Exam Prep App on Android/Web/Amazon
A Twitter List by enoumenDownload AWS machine Learning Specialty Exam Prep App on iOs
Download AWS Machine Learning Specialty Exam Prep App on Android/Web/Amazon